Duelling Dynamics
Insights into the AI-Energy Transition Nexus
Who to contact
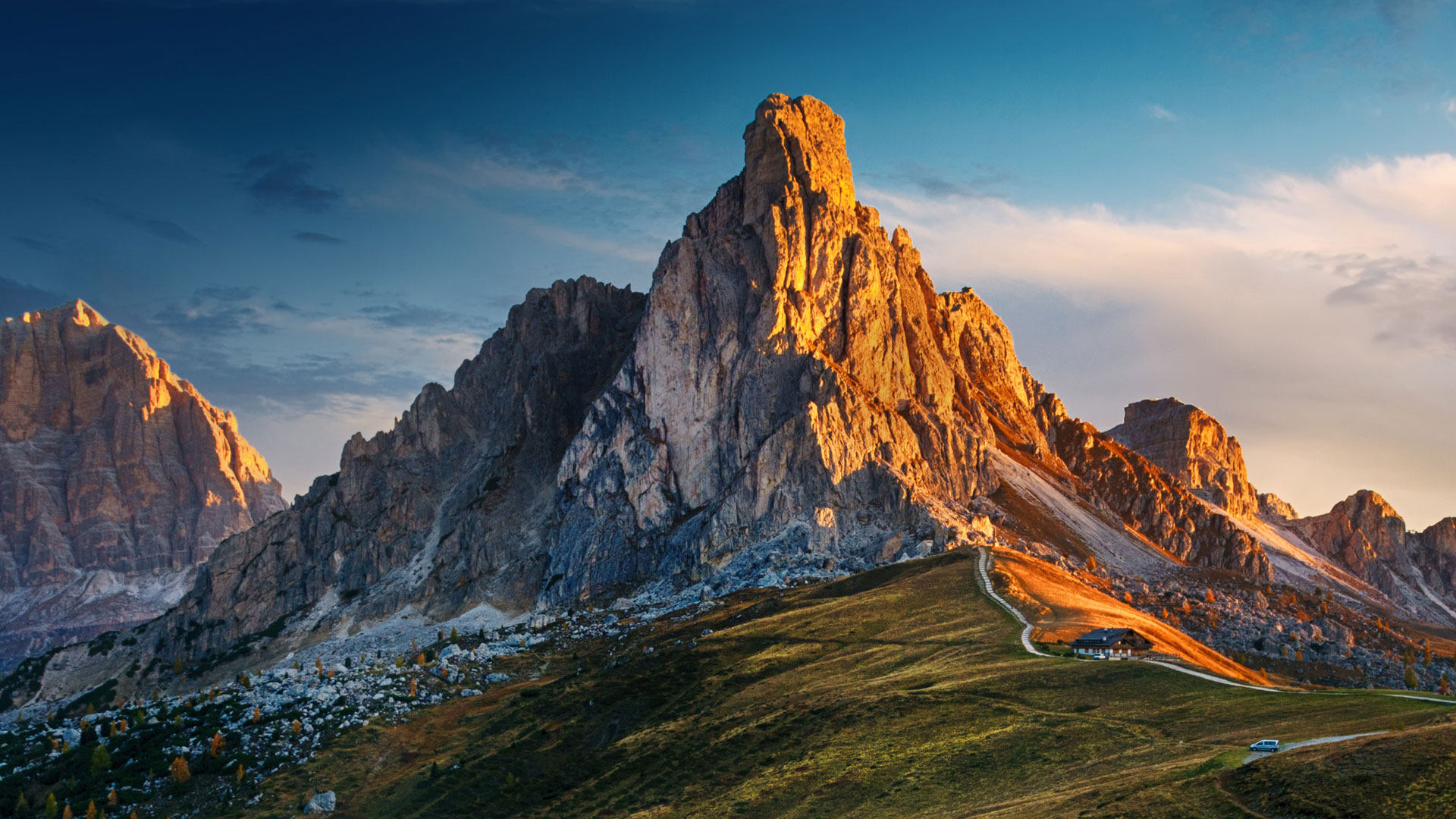
MORE FROM HORIZON SCANNING 2025
This material is provided for general information only. It does not constitute legal or other professional advice.